The physical inspection of water service lines provides a reliable and effective way of ensuring safe delivery of drinking water, especially as part of lead verification programs. However, while effective, it is also expensive — ranging anywhere between $500–$2,000 or more per property. Physical inspections are only further complicated during winter months.
To better understand the complication and provide resolution, Trinnex’s Mark Zito, Senior Solutions Consultant, and Katie Deheer, Analytics Consultant, hosted a webinar on November 9th in collaboration with Water Online, on leveraging machine learning to prioritize field verifications ahead of winter.
Machine learning requires substantial data to build a reliable model, and hence one needs an extensive inventory with little to no gaps to build a successful algorithm with good prediction accuracy and no fails. The following image shows the lifecycle of an inventory, right from gathering data to keeping it updated.

Mark explains that the process starts with recording and compiling data, often from varied sources and moves on to building the initial inventory.
Inventory development process
Field verifications provide significant help in filling in the missing gaps in the inventory. Mark explains the inventory development process:
- Step 1: Involves a desktop study, including collating GIS and property information, work orders, and meter data
- Step 2: Involves an expanded desktop study, including digitizing records
- Step 3: Involves a field study to get more data and this is where it also gets costlier
- Step 4: Involves a field investigation or verification through testing pits and vacuum excavation.
>>YOU MIGHT ALSO LIKE: Developing a Living Inventory
EPA guidance on field verifications
As per the Environmental Protection Agency’s (EPA) guidance around field verifications, utility systems must identify and track information on service line material as and when it is encountered during normal operations. Field verifications enable teams to fill gaps in inventory. If the quality of existing records is not up to date (for example, information that was compiled more than fifty years ago when the standards and methods were different), then those must be verified for accuracy.
Mark also spoke about current requirements and accepted methods for field verifications. A few accepted methods include visual inspections by customers at the point of entry (at the private side only) through CCTV (internally or externally), and visual inspections by excavation, mechanical, or hydro-vacuum. Water quality sampling, predictive modeling, and any emerging methods such as electrical or wave energy characteristics and ground penetrating radar require state approval.
Seasonal changes in field verifications
Winter brings along snow and ice, which makes intrusive outdoor investigations challenging or even impossible. Mark suggested three options to replace these:
- In-home inspections (for example, going door-to-door and using crawl spaces for investigations)
- Records research (for example, sorting through filing cabinets)
- Predictive modeling
Some more options for interior field verifications are homeowner surveys or photos submitted online (which require quality assurance) and compliance sampling inspections.
A few exterior options include test pits, vacuum excavations, and meter inspections.
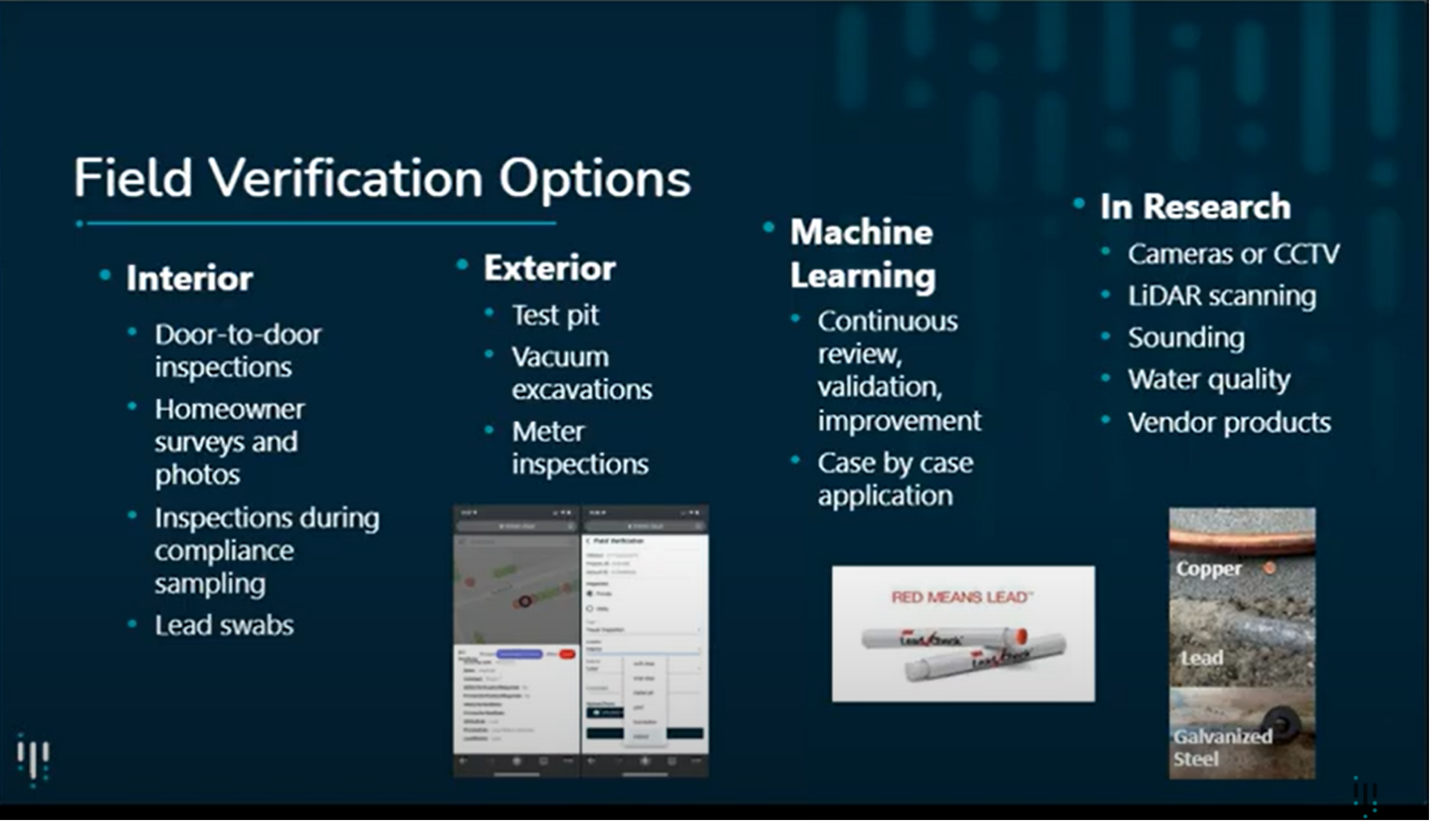
Mobile applications that tie directly into an inventory database, such as leadCAST, provide a dynamic method of keeping track of ongoing field verifications.
This on-the-go approach provides several benefits:
- Pull up required information live, when required
- Record information about the time and location where the team inspected
- Add comments/photos for additional verification
Self-reported verifications also help to close the data gap in field verifications. Self-reporting starts by setting up a secure online portal for customers to register and follow provided instructions to submit their service line material verifications. The utility team can visit the premises to follow up later. Allowing customers to self-report keeps the verification teams indoors and provides information at a lower cost.
Field verification examples
One can choose either opportunistic or proactive ways to collect information.
Opportunistic:
- Piggybacking on plumbing inspections (utilities work with inspectors of homebuyers)
- Verifying during water tap sample collection (water quality monitoring)
- Verifying during water meter or water main replacements
Proactive:
- Neighborhood canvassing; setting up signs (along the lines of, "We are coming to your neighborhood")
- Door-to-door inspections
- Mechanical excavation
Prioritizing field verifications
After discussing several ways to ease field verifications during the winter, Mark mentioned a few ways to prioritize them:
- Eliminate investigation of service lines installed post 1986 as lead lines were banned, deeming investigations unnecessary
- Perform opportunistic field work by tying into the already ongoing fieldwork; for example, at schools, playgrounds, disadvantaged neighborhoods, day cares, and so on
- Focus seasonal effort on in-home inspections rather than digging in the street in extreme conditions
The goal of field verifications is to eliminate the number of unknowns, but as Katie mentions in the webinar, there might be a more streamlined way to narrow down unknowns.
The role of machine learning in field verifications
Machine learning helps tremendously with unknowns, especially with prioritizing where to conduct field verifications. Katie explains how machine learning can suit your needs and how the gathered data can impact the machine learning model’s accuracy.
Machine learning models can approximate the number of lead service lines expected to be in the system, helping communities prioritize and plan field work efficiently, which in some cases can help secure adequate funding to replace confirmed lead service lines (we’ll talk about that more later). Mark and Katie suggest leveraging recommendations from machine learning models to target the locations where lead might exist, but to prevent biased investigations by randomizing where field work is conducted in an area.
Machine learning provides two main benefits:
- Planning and prioritizing where field inspections are most needed
- Inventory management (once the machine learning model has reached an acceptable level of accuracy)
Note that the early stage of a machine learning model is based on initial field inspections and historical records. The subsequent model iterations improve as new field verification data gets added, constantly strengthening the accuracy of the model.
Additional uses of machine learning
Katie named three additional uses of machine learning:
- Aggregate predictions to estimate the funding required to replace service lines that have low, medium, or high probability of lead. The probability ranges between 0 and 1, with zero being zero probability and one being 100 percent probability of finding lead in inspected service lines
- Provide a map, which is precise down to an individual property level, to guide field verifications
- Use early data to verify utility records, property data, and public or census data. The more the material verifications, the higher the accuracy of the model. The hit rate will increase initially with targeted field verifications and then will decrease once there is less and less lead to find
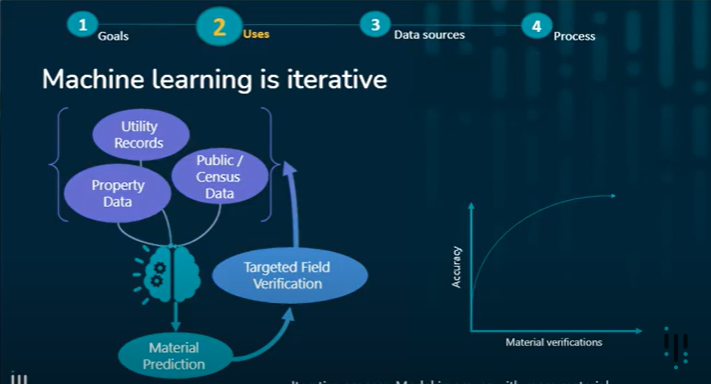
Machine learning data sources and their influence
Data sources that feed into a machine learning model come from different systems, thus requiring some cleaning. For example, housing data from sites such as Zillow and demographic data from the U.S. Census Bureau will not have the same consistency and can influence the behavior of the machine learning model. As shown in the image below, the top predictor variables among the data (that is, the most influential predictor features, which vary from community to community) are zip codes, install years, and land value.
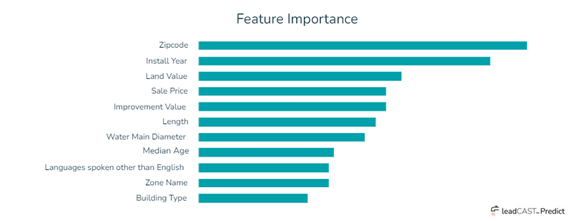
Applying machine learning to the inventory development process
Field verification data used for machine learning is split into:
- Test set: This is a small data set used to test the model on how well it does with new data
- Training set: This set involves adding data (housing, demographic, and so on) to train the model and also tests the model to see how well it does with new data
Listen more about how field verification data is used to enhance machine learning accuracy:
Once the model performs well, we can use it to predict the probability of lead for the unknown service lines.
The probability between 0 and 1 means we can classify those lines as having low, medium, or high probability of lead. The service lines with high probability will be inspected for the presence of lead. This new verification will be fed back into the model so it can learn and reach an acceptable degree of accuracy.
With any machine learning model, the hit rates will initially be high as more lead will be predicted in service lines. The hits will decrease with multiple iterations as there will be no more lead to find.
Machine learning timelines
Machine learning provides great opportunities to ease inventory development tasks but requires some initial steps to fully leverage its power. Here’s a sample schedule of how implementing machine learning could look like for you:
- December: Prepare your data for modeling
- January: Identify training data set (versus the testing data set)
- February: Run the model and identify locations to inspect (locations with the highest probability of lead with the probability being closer to 1)
- March–April: Perform targeted field investigations using the results from the machine learning model
Taking the next step with machine learning
Mark and Katie provided intelligent insights into how can accurately predict lead in service lines based on the clean inventory data. While a few challenges still exist, machine learning promises an advanced way to healthy and safe drinking water while also reducing utility teams’ efforts ahead of winters.
Watch the full webinar and gain valuable insights on using machine learning. You can also schedule a 30-minute consultation with us to review which model works best for your data situation.