Picture yourself walking through a medieval bazaar, where the air is thick with the aroma of spices and the loud noise of merchants. Each shopkeeper sells an array of goods, from mundane to exotic. Now fast forward centuries, imagine a bazaar unlike any other — a place where merchandise is invisible to the eye, yet crucial to our cities: these are water service lines.

Images created by DALL.E 3
Just as merchants in a medieval bazaar relied on meticulous knowledge of their goods to thrive, modern cities depend on a detailed inventory of water service lines to function effectively. In the bazaar, a lack of organization could lead to chaos and loss; similarly, without a well-maintained water service line inventory, cities risk water shortages, public health issues, and high repair costs. Therefore, maintaining an accurate inventory of water infrastructure is as crucial today as knowing every item in a stall was centuries ago.
Significance of Inventory Management
Take the award-wining software, leadCAST® as an example. This inventory web application serves as the innovative counterpart to the meticulous record-keeping once found in medieval bazaars. Just as merchants kept detailed accounts of their goods, leadCAST provides a comprehensive inventory of a city’s water service lines. The tool catalogs diverse data crucial for the inventory management, thus addressing the challenges posed by the lead and copper rule revision (LCRR) in identifying and replacing lead water service lines. The tool mirrors the detailed knowledge that was once necessary in medieval bazaars, but for the critical mission of preventing lead contamination today.
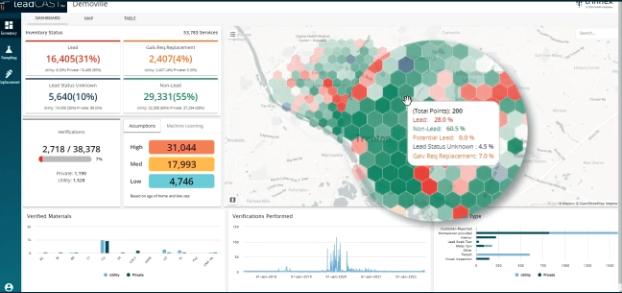
Image is adopted from the Trinnex website
Following the new guidelines, state authorities now recommend using predictive modeling, for e.g., machine learning (ML), to predict unknown lead statuses in service lines, which marks a significant shift toward data-driven decision making, national wide. In response to the guidelines, we developed leadCAST Predict, the tool that not only enhances water utilities’ ability to use ML models (by incorporating various data sources, including demographic information and historical records), but also enables a more targeted approach in identifying the presence of lead service lines. This is similar to our medieval bazaar where a shopkeeper could guess (predict) what goods are needed the most, and how soon, based on the records of sale of goods.
leadCAST Predict utilizes key data features to power its ML algorithms. These features include, the installation year of the service line, the year the property was built, and neighborhood demographics, to name a few. However, to be able to leverage ML to its fullest potential, we should ask:
- How can we maintain consistency and quality in data features across various ML models?
- What can expedite the development and deployment of ML models?
- How can we enhance data feature reusability and sharing among data science teams?
- How do we ensure data features stay accurate and up to date for effective ML models?
Feature Store
Imagine a group of medieval craftsmen, each skilled in their own right, but reliant on a shared repository of tools and materials. The feature store serves a similar purpose for leadCAST’s data scientists. It’s a centralized, managed repository that ensures features are not only reusable and accessible, but also standardized across models. This ensures that when the data scientists are predicting status of a service line, they’re using the best possible data features, leading to more accurate, reliable, and ultimately, valuable insights.

Images created by DALL.E 3
If leadCAST’s dataset is an inventory, then the feature store is akin to an advanced warehouse system, organized and filled up with valuable goods. This isn’t just any warehouse; it’s the heart of the operation, where features (the fundamental ingredients of machine learning models) are stored, cataloged, and maintained. It’s where data scientists can easily access and retrieve necessary components to craft their models.
Why is the Store Important?
In the grand bazaar of our modern world, where data is currency, the quality and organization of that data can mean the difference between success and failure. For water utilities, the stakes are safety, efficiency, and sustainability of water services.
leadCAST Predict, through its innovative use of a feature store, doesn't only keep an inventory, but also ensures that this inventory can be leveraged to its fullest potential, transforming raw data into actionable insights.

As we journey from the marketplace of the past to digital bazaars of the present, let’s leverage these purpose-built products for water utilities and ease compliance and reporting. Remember, the LCRR deadline is closer than we think.