Understanding Artificial Intelligence
At its core, AI refers to the capability of a machine to mimic intelligent human behavior. It’s about creating systems that can learn, adapt, and making decisions that usually require human intelligence. Consider two systems, Google Maps, and a vending machine.
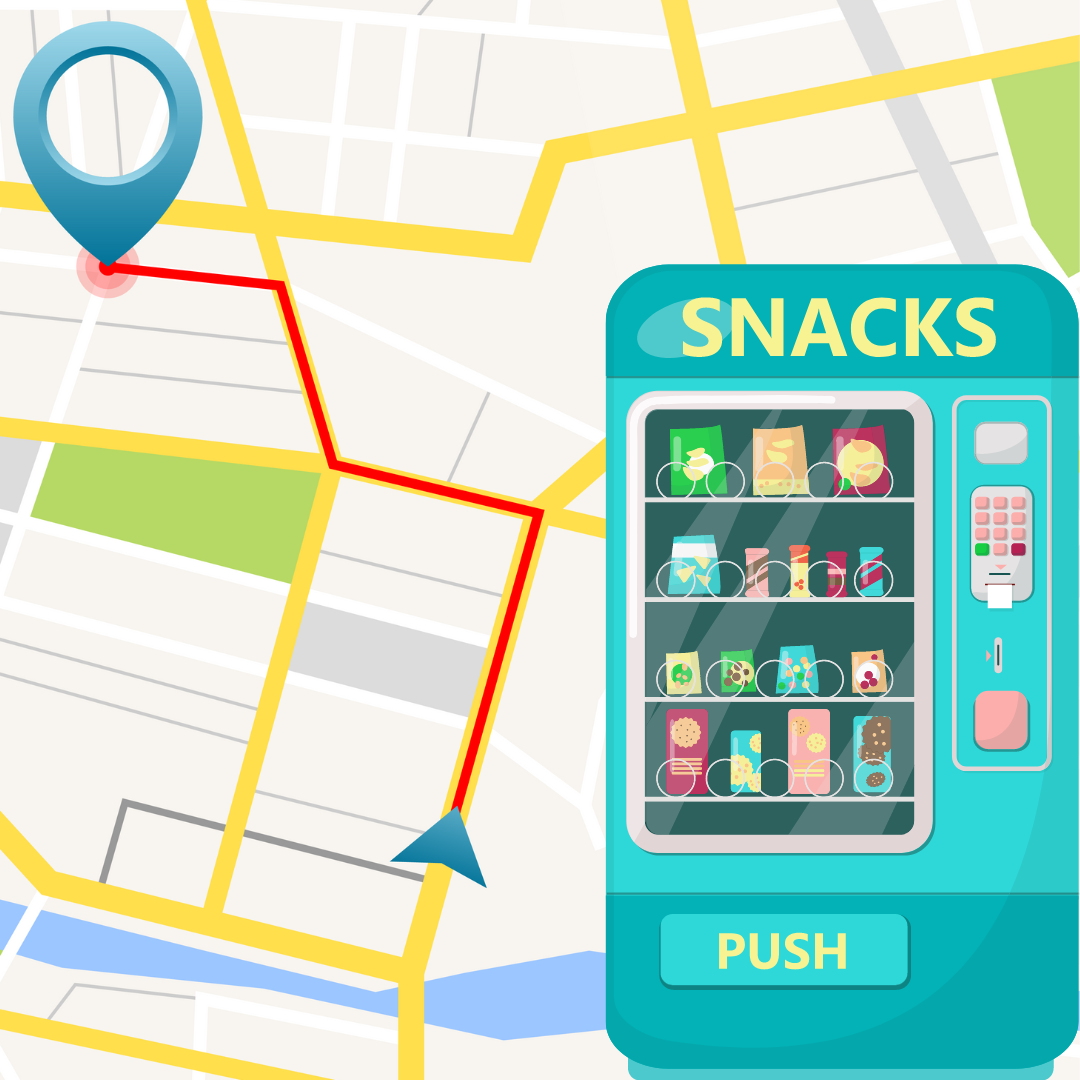
When you use Google Maps, AI helps calculate the fastest route to your destination, taking into account real-time traffic, road closures, if you drive an electric vehicle, dislike tolls, and several other factors. That’s AI at work:
We also encounter things that appear intelligent but aren’t quite AI. For example, a vending machine may seem smart because it gives you your favorite snack at the push of a button. However, it’s not AI. It’s programmed to release a product when you insert money and push the button. It doesn’t learn or adapt based on your snack preferences or how hungry you might be. This is an example of an artificially intelligent system, which, while it operates based on a set of pre-determined rules or programs, does not possess the ability to learn, reason, or make decisions beyond its initial programming.
Thin Line Between AI and Artificially Intelligent
The differentiation often lies in the depth of the intelligence. While both AI and Artificially Intelligent systems can process information and perform tasks, AI systems possess the ability to learn and improve from their experiences, a trait known as machine learning. For instance, a chess-playing AI improves its gameplay each time it plays, learning from both its wins and losses. On the other hand, an artificially intelligent chess program that only follows preset chess strategies, without learning or adapting, is merely a system that mimics intelligence. It will play the same way every time, regardless of the outcome of its previous games.
Intersection of AI and Water Utilities
With Artificial Intelligence, we can build a model for water systems that learns from the available dataset, collected from a water system. The dataset could include known characteristics such as the installation date of the service lines and perhaps the area’s historical data. Based on these known factors, AI can predict whether an unknown service line is likely made of lead or non-lead. Over time, as more data is collected and verified, this AI model can learn and improve its predictions, making it a powerful tool for decision-making.
At Trinnex®, we use such an iterative process to continuously improve service line material predictions. The figure shows the accuracy of an AI and artificially intelligent systems as the function of the percentage of unknown service lines that are verified. Accuracy is a metric used to evaluate the performance of a decision-making system. In our case, i.e., predicting lead or non-lead, the accuracy is defined as percentage of correctly identifying service lines material in a water system.
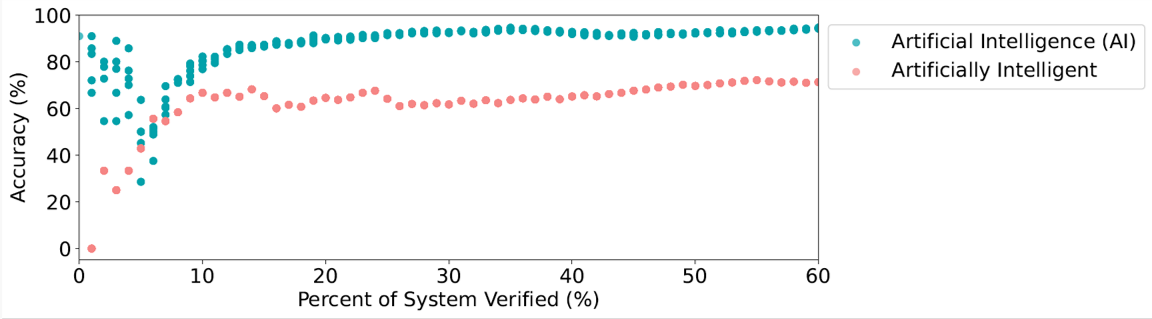
As you can see, in light of new verified service lines, the AI system adapts to new information resulting in higher accuracy, i.e., improved predictions as more service lines are verified (blue dots). On the other hand, an artificially intelligent system that just follows pre-determined rules would only classify the unknown service lines based on those fixed guidelines, without learning or adapting. For instance, we might define a rule-based decision-making process such that the service lines installed before 1950 are considered as lead (red dots). While this system might seem smart, it’s not AI. It doesn’t learn or adapt based on new data or outcomes. Instead, it applies the same rule all over again without guaranteeing improved predictions that surpasses the intelligent system. Moreover, using such a rule might not be sufficient and a human needs to incorporate more sophisticated rules incorporating other information such as service line characteristics, family income, etc. In addition, such rules might not be valid for all water utilities and could be completely re-defined.
Why is this important?
In water systems with thousands of service lines, verifying the material of each service line using conventional methods such as physical inspections are not only expensive, but also in some cases it’s not possible, e.g., a service line is located under a concrete pavement.

Using AI responsibly to predict service line materials relaxes such limitations by enabling water utilities to identify the presence of lead without the necessity of verifying all service lines at once. Instead, at Trinnex, we use a holistic approach to establish an initial optimized sample dataset to build a machine learning model. Afterwards, this model is used to provide predictions for unknown service lines and lay the foundation for next iteration of verification and model prediction improvement.
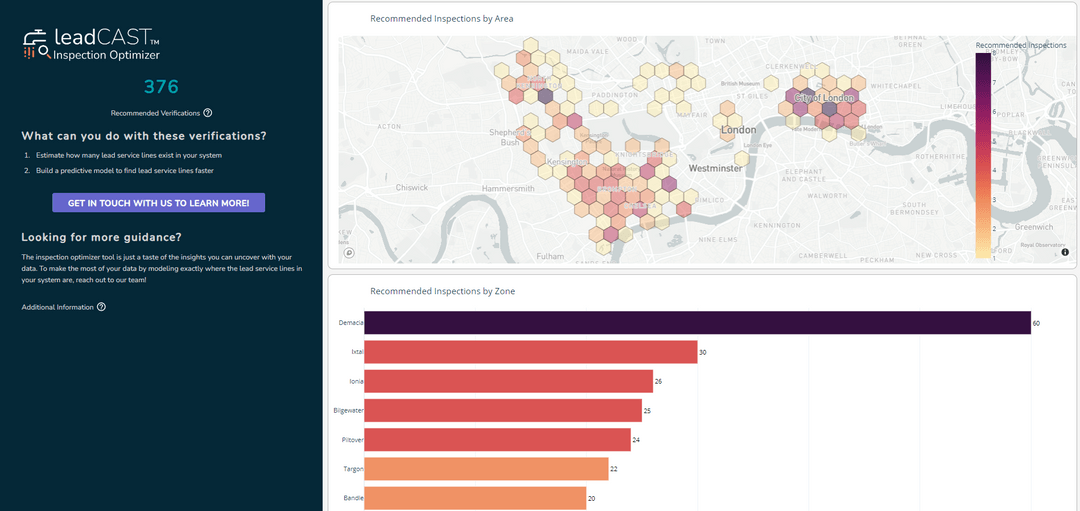