There is a lot of buzz and hype around machine learning and artificial intelligence (AI). While machine learning and AI can be powerful tools to solve practical business challenges, these kinds of techniques are not a silver bullet.
When attempting to tackle a data challenge with machine learning or AI, a couple of guiding principles can help:
- The simplest solution is usually the best, as long as it solves the problem.
- Every data problem is unique in nature and requires a creative approach.
For certain cases, the best solution might be a blend of simple logic, statistical methods, and machine learning or AI. Here’s a closer look at how Trinnex puts these principles into practice when using machine learning for anomaly detection.
Machine learning for anomaly detection in sewer flow data
Sewer flow meters provide a constant stream of information. Trying to glean insights and detect anomalies from so much data can be overwhelming.
Using a blend of statistics and machine learning, Trinnex built a solution to assist wastewater utilities with identifying sensor issues and operational problems more quickly and reliably by detecting anomalies in sewer flow data, such as:
- Sudden shifts indicative of issues such as sensor failure or blockages in the system
- More gradual changes in the data over longer periods of time, which point to issues like sensor drift or other less immediately obvious operational or environmental problems
- Differences between calibrated hydraulic models and sensor data
YOU MIGHT ALSO LIKE: How to Diagnose Hidden Problems in your Collection System Infrastructure
Determining the appropriate stage of machine learning complexity
In the initial stages of developing the solution, our data scientists tested machine learning approaches of moderate to high complexity. Some of these approaches are tried-and-true and have proven successful in detecting anomalies in a variety of different contexts.
However, after a few days of initial experimentation, we found that complex machine learning approaches performed well in some respects but poorly in others. They detected sudden shifts in flow data quite well but were not reliable or consistent at detecting more subtle anomalies like sensor drift or gradual changes over longer periods of time due to operational and/or environmental problems.
This was intriguing but not entirely unexpected.
RELEVANT BLOG POST: 3 Tips for Delivering Intuitive and Visually Compelling Analytics
Using a hybrid approach to machine learning
When faced with this challenge, our data scientists collaborated with engineers and subject matter experts to glean additional insights about the data to identify alternative methods for reliably detecting more subtle (yet impactful) anomalies in flow data.
We looked for groupings of flow meters that tend to show similar trends, reflecting similar tributary area characteristics. Accounting for these groupings and relationships turned out to be a critical success factor in building a vastly simpler “hybrid” solution for detecting more subtle anomalies than our initial attempts with more complex machine learning models.
The simple solution uses a lower complexity machine learning model in combination with statistical methods to identify when sewer flow meters start to record data that deviates from what is normal or expected. Each technique in this hybrid solution addresses unique aspects of the data, and it infuses straightforward yet critical observations about how sewer systems work.
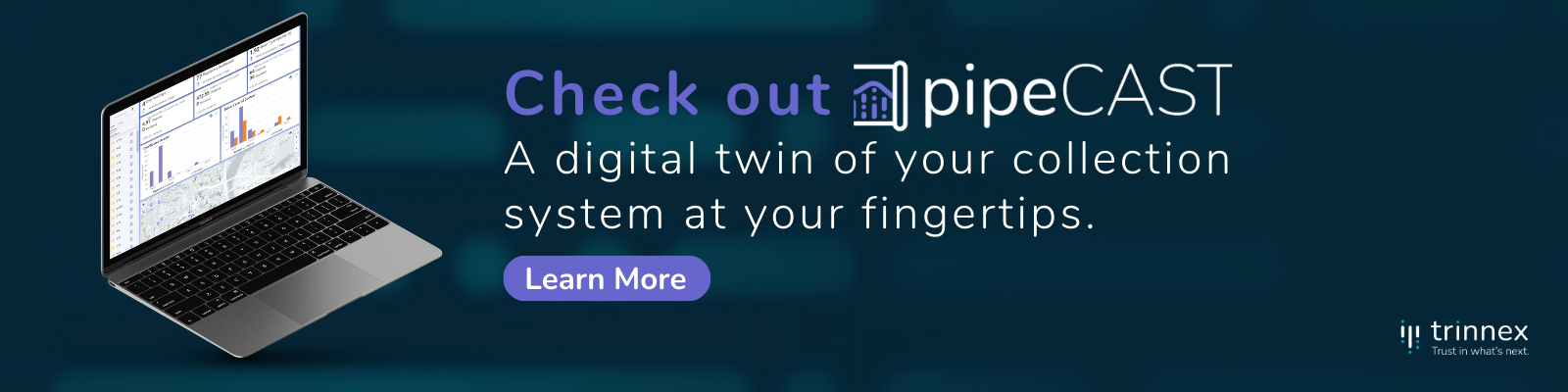
Putting the machine learning solution to the test
When we put our simplified solution to the test, we found it was highly effective at detecting subtle anomalies as well as sudden shifts in the data. In some instances, it detected issues weeks before they would have otherwise been detected during manual review or inspection.
Not only is our simple hybrid solution more effective at solving the challenge at hand compared to more complex machine learning approaches that we initially tried, but it’s also easier to understand, explain, and troubleshoot.
Want to learn more about this machine learning solution for anomaly detection?
Schedule a consultation with our machine learning experts today.